Demonstration of enhancing stock portfolio performance with AI-powered investment models

Demonstration of enhancing stock portfolio performance with AI-powered investment models
Many investment analysts have pre-selected stock titles that they trust in the long run, hold them in their portfolios, and adjust their weightings now and then. In today’s article, we will take a look at how to work effectively with your stock universe and achieve better performance by leveraging machine learning and AI-powered stock-picking models (Learn more in our article Stock Analysis with The Power of AI).
According to our approach, you only hold stocks that you or your analysts believe in. However, you select only a portion of them for your portfolio to achieve a higher CAGR, a lower Drawdown, or any other indicator that might be important to you.
The process of creating AI-powered Investment Models
To illustrate the case, we took the AMC (Actively Managed Certificate) of one of the portfolio management interested parties. The instrument is composed of 18 shares (We cannot display all of them due to the protection of trade secrets. However, for instance, the shares of Nvidia Corp., Palantir Technologies Inc., Mobileye Global Inc., or Intuitive Surgical Inc. are included in the portfolio) and cash.
Later on, we looked at its performance[i] from 26 Jan 2018 to the end of 2023, i.e. over the last 6 years.
In the next step, we trained 4 investment models on this 18-stock universe to select stocks for the portfolio.First, let’s take a general and very simplified look at how investment models can be trained.
Models used
- Lasso: linear regression using L1 regularization to prevent overfitting. Read more: https://en.wikipedia.org/wiki/Lasso_(statistics)
- Ridge: linear regression using L2 regularization to prevent overtraining. Read more: https://en.wikipedia.org/wiki/Ridge_regression
- Elasticnet: linear regression using both (L1 and L2) types of regularization. Read more: https://en.wikipedia.org/wiki/Elastic_net_regularization
- Xgboost: an algorithm based on the principle of decision trees. Read more: https://en.wikipedia.org/wiki/XGBoost
- Averaging: the model simply averages the results of all the above models, thereby increasing the robustness and resilience of the final estimates.
According to these models, we modeled sample portfolios with 6, 7, or 8 selected stocks in the portfolio.
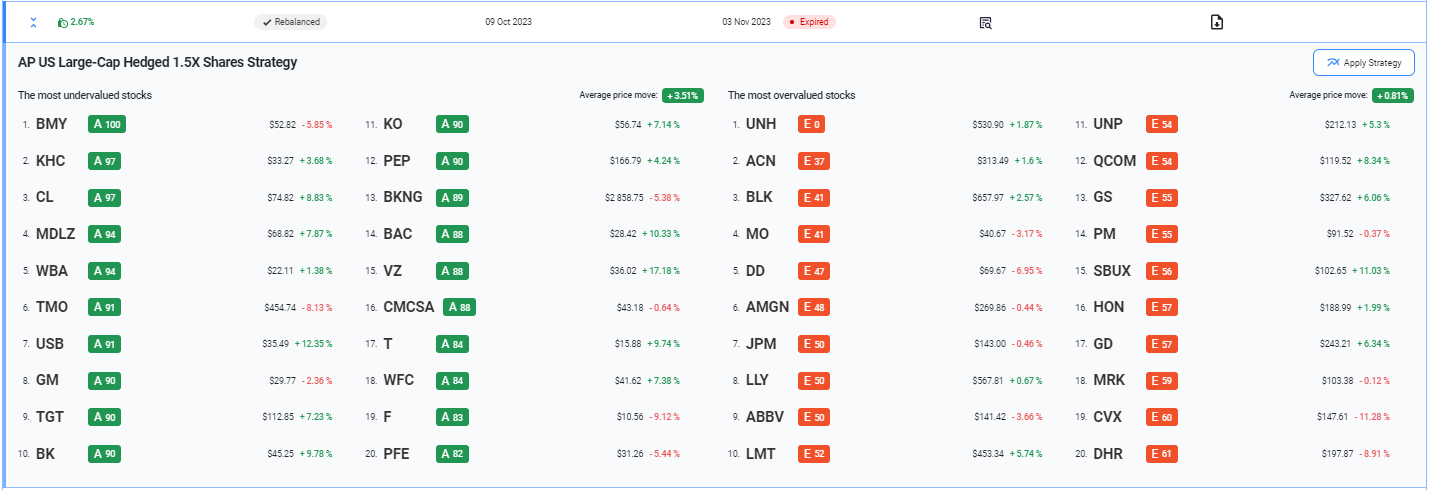
Strategies used
- Long only: The strategy selects N stocks (6, 7 or 8) with the highest growth potential as estimated by the model. The hypothesis of the strategy is that the model can generate and estimate the scoring for each firm, where higher scoring means higher future growth potential (in line with the theory of factor models).
- Short only (used for hedging): the purpose is to compare performance with Long only (the “best” ones as estimated by the model). If the model works correctly, the Short only strategy should underperform the Long only.
- Long/short: A market-neutral strategy where the hypothesis is that returns, unless they are random (i.e. the model can distinguish between “good” and “bad” stocks), are greater than 0 (because: returns long > short). Long/short portfolios have been constructed using leverage, specifically leverage of 1.5, where we borrow 50% extra capital. 2/3 (1.0) of the portfolio is invested in Long positions and 1/3 (0.5) is hedged within Short positions.In the test, the portfolio was rebalanced once a month. That means, that once a month, according to the individual investment models, a score was calculated for all shares in the pool. The stocks were sorted from the share with the highest value to the share with the lowest value. 6, 7, or 8 shares were subsequently included in the portfolio (in the case of a Long/short portfolio, 12, 14, or 16 shares). The portfolio was always equally weighted, i.e. equal capital was invested in each share.
We got interesting results.
Results of AI-powered Stock-Picking Models
- Rating of the AMC: more volatile, dynamic firms, often in the technology sector, are present in the assessed pool. Over the period under review, performance is above average compared to, for example, the S&P 500. The universe as a whole has performed at +194% for the period considered. That is, of the $100,000 invested, $294,000 would have been at the end of the period.
- Tested models rating: using our approach it was possible to significantly improve the performance of the portfolio. For instance, using the xgboost model with 7 stocks in the portfolio, the performance of the Long only portfolio would be +292% ($392,000), while having a lower maximum drawdown than the entire 18 stocks.
Let’s take a closer look at the results of the stock pool and the individual AI-powered models. We present the performance of all the indicators we track for the model that achieved the highest equity value, followed by the Final-Equity for the Long only portfolio for all assessed models.
Xgboost - 7 stocks | LongOnly | LongTheShort | LongShort | Universe |
---|---|---|---|---|
Final-Equity | 3,9180120 | 2,5162511 | 2,4699535 | 2,9494334 |
Volatility | 0,3182914 | 0,2807583 | 0,2042959 | 0,2865860 |
Sharpe | 0,9127866 | 0,7029999 | 0,8899390 | 0,8011896 |
Alpha-Universe | 0,0440671 | -0,0181716 | 0,0448920 | 0,0000000 |
Alpha-SP500 | 0,2831596 | 0,1936984 | 0,1764390 | 0,2257429 |
Beta-Universe | 1,0733792 | 0,9387680 | 0,5964724 | 1,0000000 |
Beta-SP500 | 0,1299554 | 0,0655740 | 0,0951233 | 0,0689188 |
CAGR | 0,2596111 | 0,1687808 | 0,1651181 | 0,2005837 |
Max-Drawdown | -0,4830035 | -0,4892133 | -0,3312657 | -0,4899071 |
Average-Drawdown | -0,1357781 | -0,1395648 | -0,0797468 | -0,1400689 |
Win-Rate | 0,5857143 | 0,6142857 | 0,5714286 | 0,6000000 |
Skewness | 0,1535893 | -0,3610750 | 0,4846793 | -0,2819573 |
Kurtosis | -0,0065122 | -0,0386901 | 0,3209663 | -0,0469967 |
VaR95 | -0,1181847 | -0,1094379 | -0,0658765 | -0,1302112 |
CVaR95 | -0,1599593 | -0,1616658 | -0,0894731 | -0,1647018 |
Max-Drawdown-Duration | 29 | 26 | 29 | 27 |
Calmar | 0,53749316 | 0,345004535 | 0,49844592 | 0,40943207 |
Sterling | 1,07030365 | 0,574743953 | 0,83062673 | 0,73002655 |
Sortino | 1,93959219 | 1,268539907 | 2,04989193 | 1,51220602 |
Information on the various investment metrics we use in building investment strategies can be found in our ‘Configuration of investment strategies‘ series of articles. Would you like to take the same approach to managing your portfolio? Take advantage of our AI-powered Factor Investing Software/ models.
Model | Lasso | Ridge | Elasticnet | Xgboost | Averaging | Universe |
---|---|---|---|---|---|---|
6 stocks | 2,474745 | 3,464498 | 2,608561 | 3,189853 | 3,014739 | 2,949433 |
7 stocks | 3,401176 | 3,262271 | 3,079037 | 3,918012 | 3,224239 | 2,949433 |
8stocks | 3,428182 | 3,771866 | 3,237451 | 3,821567 | 3,256669 | 2,949433 |
Invest in the forces that move stock prices
Leverage the power of factor investing with revolutionary software
[i] To make things simpler, we have set each stock to have the same weight in the portfolio at all times; in real life, analysts adjust the weights dynamically.